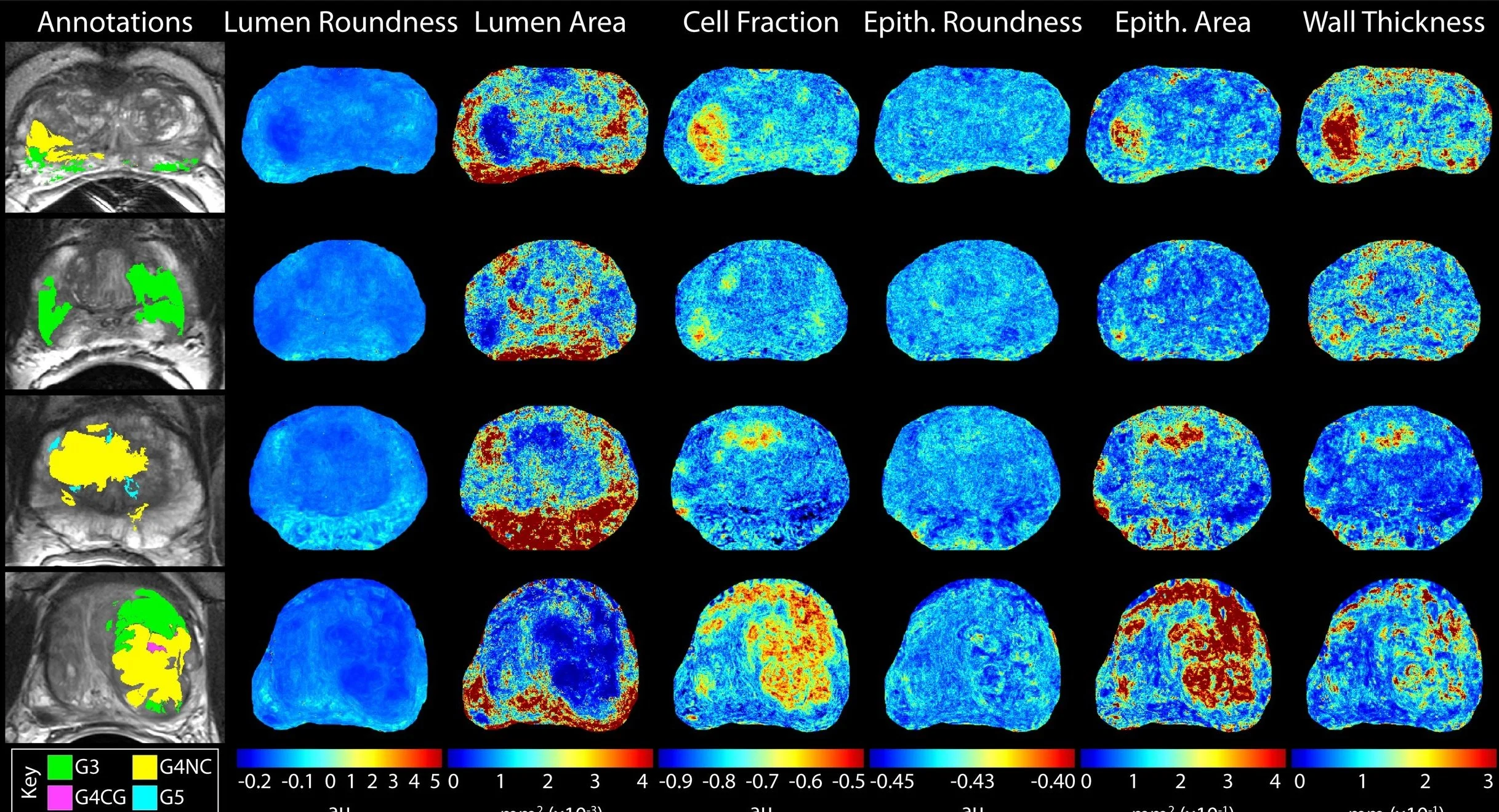
Prostate Cancer Radio-Pathomics
Prostate Cancer
Relevance
Prostate cancer (PCa) is the leading male cancer diagnosis in the United States, accounting for 30% of all new male cancer diagnoses. PCa may first be detected through abnormal digital rectal exam results or after elevated prostate specific antigen (PSA) levels are detected. Patients may then undergo multi-parametric magnetic resonance imaging (MP-MRI), which is assessed using the Prostate Imaging Reporting and Data System (PI-RADS). Needle biopsies may be collected if suspected tumor regions are found on the MRI. This tissue is graded using the Gleason grading system, assigning a score based on glandular patterns present. If clinically significant cancer is detected, the patient may undergo radical prostatectomy, complete removal of the prostate and seminal vesicles, amongst other treatments such as chemotherapy and/or radiation. While the 5-year survival rate of PCa is nearly 100 percent, upwards of 20% of men will experience biochemical recurrence (BCR), detected through elevated PSA levels post-surgery. A potential flaw with current standard of care is the overtreatment of patients with low-risk disease. PCa treatment can cause impotence, incontinence, and infection which can hinder patient wellness. It is critical to determine novel imaging tools to precisely detect cancer presence and predict metastatic potential to give patients the best treatment possible and prevent further hospitalizations, procedures, or lifelong side effects from unnecessary treatment.
Our Work
Our work uses tissue samples collected following radical prostatectomy aligned to MR imaging acquired prior to treatment to determine the relationships between radiological signatures and the underlying pathology of prostate cancer lesions. Specifically, we use clinical MRI coupled with machine learning-based predictive models can distinguish regions of aggressive tumors and patients with high metastatic potential by using surgical tissue coupled with pathological cancer annotations as ground truth.
Our most recent work in prostate cancer include determining whether digital slide scanners impact tissue-based features, comparing machine and deep learning models to automatically annotate Gleason patterns on digital histology, quantifying histological features predictive of biochemical recurrence, using radiomic features to predict cancer presence and BCR. Our newest work involves creating radio-pathomic maps of prostate cancer using models of MRI intensity to predict histological features, and then these features to predict tumor location. Keep your eyes peeled for our upcoming publications using these models!
Duenweg SR, Brehler M, Lowman AK, Bobholz SA, Kyereme F, Winiarz A, et al. Quantitative Histomorphometric Features of Prostate Cancer Predict Patients Who Biochemically Recur Following Prostatectomy. Lab Invest. 2023;103(12):100269. Epub 20231026. doi: 10.1016/j.labinv.2023.100269. PubMed PMID: 37898290; PubMed Central PMCID: PMC10872376.
Duenweg SR, Bobholz SA, Barrett MJ, Lowman AK, Winiarz A, Nath B, et al. T2-Weighted MRI Radiomic Features Predict Prostate Cancer Presence and Eventual Biochemical Recurrence. Cancers (Basel). 2023;15(18). Epub 20230906. doi: 10.3390/cancers15184437. PubMed PMID: 37760407; PubMed Central PMCID: PMC10526331.
Duenweg SR, Bobholz SA, Lowman AK, Stebbins MA, Winiarz A, Nath B, et al. Whole slide imaging (WSI) scanner differences influence optical and computed properties of digitized prostate cancer histology. J Pathol Inform. 2023;14:100321. Epub 20230704. doi: 10.1016/j.jpi.2023.100321. PubMed PMID: 37496560; PubMed Central PMCID: PMC10365953.
Duenweg SR, Brehler M, Bobholz SA, Lowman AK, Winiarz A, Kyereme F, et al. Comparison of a machine and deep learning model for automated tumor annotation on digitized whole slide prostate cancer histology. PLoS One. 2023;18(3):e0278084. Epub 20230316. doi: 10.1371/journal.pone.0278084. PubMed PMID: 36928230; PubMed Central PMCID: PMC10019669.
Ruchti A, Neuwirth A, Lowman AK, Duenweg SR, LaViolette PS, Bukowy JD. Homologous point transformer for multi-modality prostate image registration. PeerJ Comput Sci. 2022;8:e1155. Epub 20221201. doi: 10.7717/peerj-cs.1155. PubMed PMID: 36532813; PubMed Central PMCID: PMC9748842.
Duenweg SR, Fang X, Bobholz SA, Lowman AK, Brehler M, Kyereme F, et al. Diffusion Restriction Comparison between Gleason 4 Fused Glands and Cribriform Glands within Patient Using Whole-Mount Prostate Pathology as Ground Truth. Tomography. 2022;8(2):635-43. doi: doi:10.3390/tomography8020053. PubMed PMID: 35314630; PubMed Central PMCID PMC8938782.
McGarry SD, Brehler M, Bukowy JD, Lowman AK, Bobholz SA, Duenweg SR, et al. Multi‐Site Concordance of Diffusion‐Weighted Imaging Quantification for Assessing Prostate Cancer Aggressiveness. Journal of Magnetic Resonance Imaging. 2021. doi: 10.1002/jmri.27983. PubMed PMID: 34767682; PubMed Central PMCID PMC9095769
McGarry SD, Bukowy JD, Iczkowski KA, Unteriner JG, Duvnjak P, Lowman AK, et al. Gleason probability maps: A radiomics tool for mapping prostate cancer likelihood in mri space. Tomography. 2019;5(1). doi: 10.18383/j.tom.2018.00033. PubMed PMID: 30854450; PubMed Central PMCID: PMC6403022
McGarry SD, Hurrell SL, Iczkowski KA, Hall W, Kaczmarowski AL, Banerjee A, et al. Radio-pathomic Maps of Epithelium and Lumen Density Predict the Location of High-Grade Prostate Cancer. International Journal of Radiation Oncology Biology Physics. 2018;101(5). doi: 10.1016/j.ijrobp.2018.04.044. PubMed PMID: 29908785; PubMed Central PMCID: PMC6190585